The Matsuo Laboratory is conducting research with the vision of ” creating intelligence.
In this article, we asked Masahiro Suzuki, Project Assistant Professor at Matsuo Lab, about the “world model,” which is an important research theme in the creation of intelligence.
(The interview with Mr. Suzuki will be delivered in two parts, Part 1 and Part 2. Part 2 is here )
A world model that provides an “intuitive” understanding of the world is essential in the realization of true intelligence.
Before I ask you to explain the “world model,” please tell me why the Matsuo Institute is promoting research on the “world model.
This is because the Matsuo Lab has a vision of “creating intelligence,“and the world model is essential to the realization of true intelligence.
The world model, which will be explained in more detail later, refers to “a model that acquires the structure of the external world through learning based on observed information obtained from the external world (the world).
We believe that the world model is the base of intelligence, upon which various intelligent functions can be realized.
As Dr. Matsuo used to say,the world model is the “intelligence of a child.
In other words, itinteracts with the external world and understands “intuitively” what the world is like without being taught by parents.
Once this is done, we can finally begin to create “adult intelligence” like ours, i.e., artificial intelligence that achieves advanced intellectual behavior such as solving math problems and cleaning up.
Looking back at intelligence research to date, in classical artificial intelligence (also known as Good Old Fashioned AI; GOFAI),
From the beginning, it tried to achieve advanced “adult intelligence” (smart intelligence) such as search and reasoning.
The reason why it failed is because these intelligences were “ignorant” about the “world”.
Therefore, algorithms that worked very well on the computer often did not work at all in the real environment.
This may be sufficient if we are satisfied with intelligence that works only on the computer, but in order to achieve artificial intelligence that works in our real world and supports us,
However, in order to achieve artificial intelligence that can operate in the real world and support us, we must first understand the world in our own way, i.e., acquire a model of the world.
Prediction” and “inference” enable efficient control learning.
Please elaborate on what the global model will allow us to do.
As mentioned above, the world model is “a model that acquires the structure of the external world through learning based on observed information obtained from the external world (the world).
Observation here refers to various types of information obtained from the outside world, including images, sounds, and documents. The important point of the world model is to create a large-scale model of the external world by learning from these observations.
By having a world model, two main things can be achieved : forecasting and inference.
The first, forecasting, is the prediction of future or unknown observations from current observations.
Example: “Predicting” that a glass will break when it is knocked to the ground.
For example, it can predict the entire room from a cutout image of a part of the room, or predict where an object will fall a few seconds later based on how it falls.
It can also be linked to actions to make predictions that have not happened in reality, such as “this is what will happen if I act this way” (generally, when it is linked to actions, it is often called a world model).
The second type of inference is the acquisition of “representations” (* called latent variables or state representations) of the external world from observations from the external world.
E.g., “infer” the whole desk by looking at a part of the desk.
A representation here is like a compact representation of the entire outside world by compressing higher-dimensional observations such as images and sounds in the spatial and temporal directions.
For example, an observation of an image of part of a room can acquire a conceptual representation of the entire room, such as “someone’s room”. Acquiring such a representation also allows us to make quicker and higher-performance predictions within the representation.
When we predict or plan for the future, we do not always consider changes at the observational level.
For example, when solving a math problem, we do not think about how we will move our arms and hands to write the letters on the answer sheet. When we play shogi (Japanese chess), we do not think about the detailed angles and positional changes of the pieces visually in order to predict the movement of the opponent’s pieces (or even the detailed movement of the opponent’s moves). Instead, we think of conceptual representations such as “mathematical formulas” or “pieces and their positions on the board” that are brought to mind by the world model from visual information, and then we think of operations and changes in those concepts.
In other words, being able to reason about a good representation for each task (mathematical equations for mathematics, pieces and their positions on the board for chess) helps us to better predict and plan for the future.
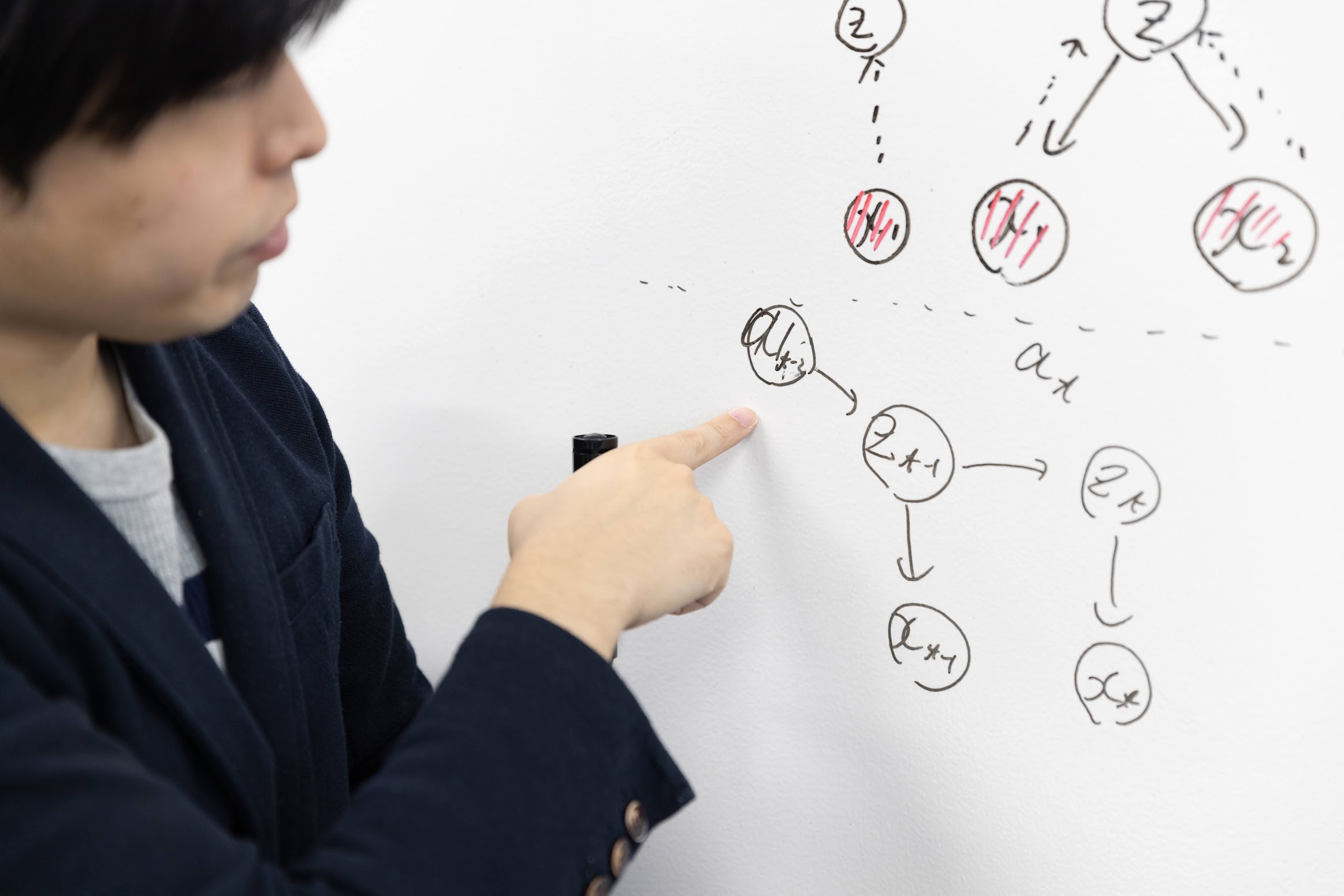
What are the benefits of adopting a global model?
Obtaining a model of the world in which such predictions and inferences can be made has a variety of advantages for artificial intelligence.
First, by having a freely predictable model of the world at our disposal, we can learn as many times as we like on a simulation with a world model when learning to control a robot or other object that may fail if moved too far . This can be likened to image training for humans. We can also infer good representations and then plan and learn how to control them to further improve their performance.
Furthermore, learning a world model by deep learning means acquiring a “differentiable world model”. This makes learning of control very efficient.
The above concept of a world model has actually been around for some time.
In the case of control with action, they have long been considered as internal models, and in cognitive psychology they have been considered as mental models.
However, it is only recently, with the advancement of deep learning research, that these can actually be learned directly from images and documents to acquire representations.
High-profile research in the world. Future challenges are efficient studies with limited scope.
Is there a high level of public interest in the global model?
The term “world model” is now commonplace. It has been rapidly studied in the area of deep learning in recent years and is a key topic for the future of artificial intelligence, as one of the major experts in artificial intelligence research, Professor Yann LeCun, has pointed out, ” It is an important research for the next AI.
Since DeepMind, Google Brain, and others are also focusing their research on world models and have published many papers, we expect the social impact of this technological development to be very large.
What are some of the challenges you face as you work on your global model?
One of the major challenges of global modeling is how to learn a high-performance model that can make predictions with a limited range of observations.
In general, instead of simply modeling “what the world would be like,” we model “what we would do to the world” by using information such as actions and perspectives as input. Since it is impossible to model the entire real world, we aim for efficient learning by limiting the scope.
Recently, large-scale models such as foundational models have emerged that can produce very well performing outputs for arbitrary inputs (prompts), and applying these models to learning about the environment may be solving these learning-related problems. There are a number of other issues that remain to be solved essentially, such as how to infer time-series information, how to integrate it with symbolic information, and how to build world models that use multiple types of observations.

From knowledge systematization to robot applications. Promotion of global modeling research at Matsuo Lab.
How does the Matsuo Lab promote global model research?
In the global model, the Matsuo Lab not only conducts basic research, but also offers lectures for human resource development with the aim of systematizing knowledge, and conducts research on robots to realize intelligence in the real world.

In terms of systematization of knowledge, we launched the ” World Model Simulator Endowed Chair ” in FY2021 to promote research and development of world models and educational activities, and launched (probably) the world’s first lecture that allows students to learn about world models in a systematic way.
In addition, we are also engaged in various other activities to promote world model research, such as organizing an organized session on world models at the national conference of the Japanese Society for Artificial Intelligence, holding a world model workshop at NEURO, and special issues on world models in several journals.
In terms of robotics research, we are working toward the application and implementation of the world model in robotics, including the construction of test beds for cleaning up robots and flexible object manipulation,
We aim to develop learning methods that can adapt to diverse environments and tasks, and to systematize methods for building scalable service robot systems.
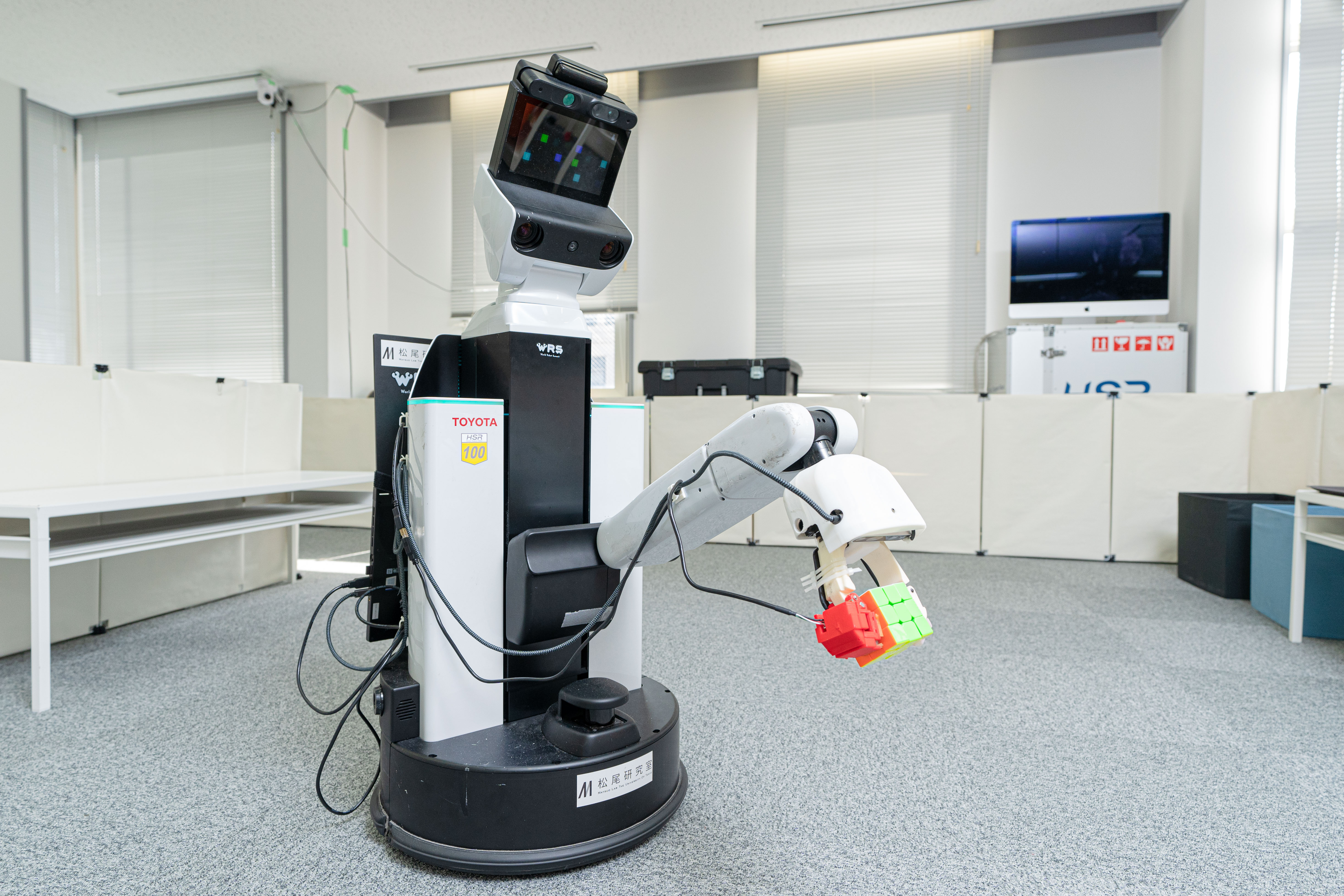
In this way, Matsuo Lab is researching and promoting the “World Model” to realize our vision of “Creating Intelligence”. If you are interested in our work in any way, please contact us for a casual interview.
Masahiro Suzuki / Project Assistant Professor, Matsuo Laboratory, The University of Tokyo
career
- Mar 2015 Completed Graduate School of Information Science and Technology, Hokkaido University
- Mar 2018 Completed Graduate School of Engineering, The University of Tokyo
- Apr 2018-Jul 2020 Specially Appointed Researcher, Graduate School of Engineering, The University of Tokyo
- Aug. 2020- Specially Appointed Assistant Professor, Graduate School of Engineering, The University of Tokyo
- Other concurrent positions︓Technical Advisor, Denso Corporation; Visiting Researcher, Ritsumeikan University
(one’s) (special) field (of study)
- Transfer learning, deep generative models, multimodal learning
Awards
- Information Processing Society of Japan Paper Award, Information Processing Society of Japan Journal Special Prize, and
- Student Incentive Award, WBAI Incentive Award, National Conference on Artificial Intelligence, Japan
- Awarded by the Dean of the Graduate School of Engineering, The University of Tokyo, for his research.
Other Activities
- Lecturer of “Fundamentals of Deep Learning,” “Deep Generative Models,” “World Models and Intelligence,” etc.
- Supervised translation (compilation of translations) and assigned translations of “deep learning” and “reinforcement learning
==============================================
In the latter half of the article, we will tell you about the research environment and the type of person we are looking for at Matsuo Lab.
Please read it as well.
Applications for Research Scientist, Assistant Professor, and Lecturer positions at the Matsuo Lab are available here.
Masahiro Suzuki will be a speaker at “The Global Model: Overview and Potential Applications” (Tuesday, December 13, 2022). Click here for details .
The Robotics Team is holding an advent calendar!