
Research on “transfer learning,” the application of knowledge acquired in the past, is progressing.
This issue features an interview with Dr. Wataru Kumagai, who has been conducting research in this area and recently joined Matsuo Lab as a specially-appointed assistant professor on August 1.
Transition learning is something that we humans do as a matter of course in the process of growing up and in our daily lives,” says Dr. Kumagai. We asked him why he is focusing on transfer learning among the general-purpose AI that is gradually being discussed.
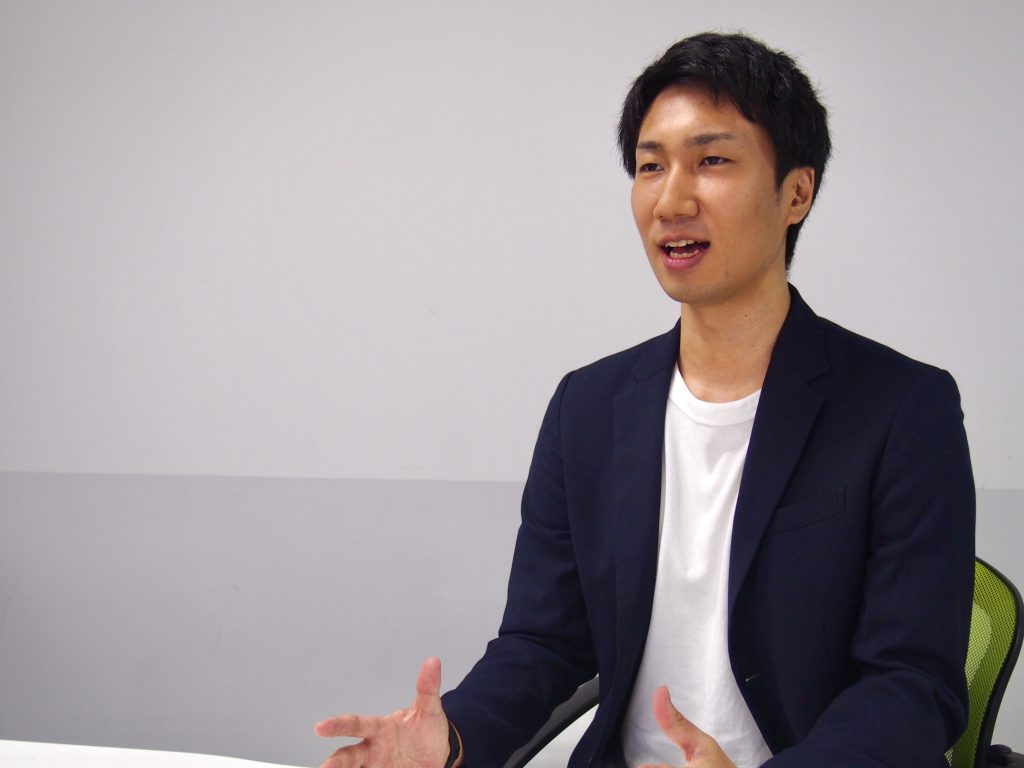
「問題を解く」とはどういうことか?
As an undergraduate, I studied mathematics. In the process, I began to think about what it means to solve a problem, and I thought, “Why don’t we create something smarter than humans? Not only in mathematics, but I thought that if we could create an artificial intelligence that is smarter than humans, it would be able to solve all the problems in the world.
At the time, I did not understand the methods used to achieve this, so I majored in mathematics for my master’s degree as well, hoping to advance in a basic direction for the time being. However, my interest in AI did not fade as I progressed from master’s to doctoral studies, and in fact, I studied machine learning intensively in the latter half of my doctoral program, partly due to the excitement of the tertiary boom. Since then, I have deepened my AI research through several positions.
I joined the Matsuo Lab not only because it is one of the best AI laboratories in Japan, but also because many of its members study general-purpose AI.
In AI research, the field of specialized AI, represented by AlphaGo, for example, has been leading the way. Since research on general-purpose AI, which is my main interest, has not yet progressed that far, it is a big plus for me to be able to exchange information on this field. In addition, since each member of the group is conducting a wide range of research, it is easy to follow the latest results in the rapidly developing field of AI research, which is also attractive.
過去に得た知識を応用する「転移学習」
My main research theme is “general versatility of AI” as a major topic. Among them, I am particularly interested in “transition learning” and am studying it.
Transfer learning is the area of “learning how to apply knowledge gained in the past to current problems. This is something we do naturally as we grow up. For example, multiplication applies the concept of addition. For example, multiplication is based on the concept of addition: “2 x 3 means that there are three 2s. Humans can generalize or conceptualize knowledge and experience and use it as wisdom that can be applied to the next occasion.
If this transfer learning were not possible, it would be very difficult for humans to survive. Similarly, we believe that the implementation of transfer learning is essential to create a general-purpose AI that can be applied.
In addition, we are also studying “continuous learning,” an evolving field of transition learning. We are particularly interested in functions related to memory, which is also an important area for general-purpose AI. This is a model in which the information acquired is made flesh and blood, and then maintained. Generally, AI is thought to be all-powerful, but it is very bad at memory, and when it learns something new, it quickly forgets its previous knowledge. Storing what it has learned and retrieving and using it when needed is another thing that is natural to humans but difficult for AI.
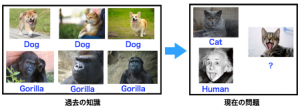
A model of transfer learning (image). If we learn the characteristics of dogs and gorillas, we can apply their wisdom to distinguish between cats and humans.
「適当に」「よしなに」への対応をアルゴリズムにするには
In order to create an AI that is at least as capable as a human, I thought about the most important part of the human “process of becoming smarter,” and came up with the idea of “accumulating past knowledge and applying it to the present.
Specialized AI can solve highly challenging problems in that field, but AI has not yet paved the way for building on basic knowledge and applying it. It is extremely difficult to put into an algorithm the ability to understand and respond appropriately to instructions such as, “Clean up the messy room appropriately,” or “Reply to this email, good day.
We are probably only at the third station before we can create a general-purpose AI that can do such things and is capable of transfer learning. We have a long way to go, butattention is growing, for example, with transition learning being featured at aconference where Dr. Matsuo spoke the year before last (New Economy Summit 2018 (NEST2018)* hosted by the New Economy Alliance).
Specifically, it may be possible to create a model that can be used universally by having medical data learned across each hospital.
If there is a large amount of usable medical data, it is possible to construct a model that can infer and diagnose certain diseases through deep learning. However, the amount of data is not sufficient for individual hospitals, and data cannot be taken out across hospitals due to privacy issues. Also, each hospital may have many elderly patients or mainly pediatric patients, and the number of patients may vary depending on whether the hospital is located in the city center or in the region.
We believe that transfer learning, including the allowance for such fluctuations, will make possible an AI that does not take data out of each hospital, but only acquires knowledge and becomes smarter and smarter as it repeatedly goes to the next hospital. …… If you think about it, the process of residents gaining experience at each hospital is also transfer learning, since they do not take data with them. It is also important not to believe too much in past examples.
知識や記憶を応用できるAIの構築に向けて
In the future, I would like to further solidify the basic theory regarding general-purpose AI. Currently, various methods and architectures are being proposed for building general-purpose AI, but I feel that there is still insufficient discussion on theoretical aspects such as “why it behaves intelligently” and “what intelligence is in the first place.
The question of “what is intelligence?” can be discussed philosophically in some directions, and I am personally interested in this question, but for my research, I will delve into it from an engineering perspective. Our goal is to extract the elements necessary to “actually program and build a general-purpose AI” and reproduce them in engineering terms.
Recently, “general-purpose artificial intelligence research groups” have been activated and a number of specialized books have been published. AI, which has human-like intelligence and intellect and can be applied, used to give the impression of a pipe dream, but concrete discussions have been progressing. In this trend, we would like to deepen transition and continuous learning.
若手研究者やAI研究に興味がある学生へメッセージ
The area that AI research deals with is very broad, and there is still room for people from any background to do research, depending on their motivation. I was in a different field until my PhD program, so it’s not too late to start. If you are interested, please jump in.
Profile]Wataru Kumagai
学部と修士課程では数学を専攻。2013年 東北大学大学院 情報科学研究科 博士課程修了。名古屋大学 学振PD、神奈川大学 工学部 特任助教、理化学研究所 革新知能統合研究センターの研究員を経て、2020年8月に松尾研に特任助教として参画。