Prof Danushka, who works for Amazon Inc, visited the Matsuo Lab on Wednesday 31 July.
Prof. Danushka belonged to Ishizuka Laboratory as well as Prof. Matsuo, and has had a long-standing relationship with him. Because of this relationship, he has given a lecture every year at our lab.
On the day of the lecture, nearly 100 people attended, including not only researchers and students from the Matsuo Lab but also online participants such as On the day of the lecture, nearly 100 people attended, including not only researchers and students from the Matsuo Lab but also online participants such as the students attending the lectures, and he gave a lecture on the theme “Air-reading LLM”.

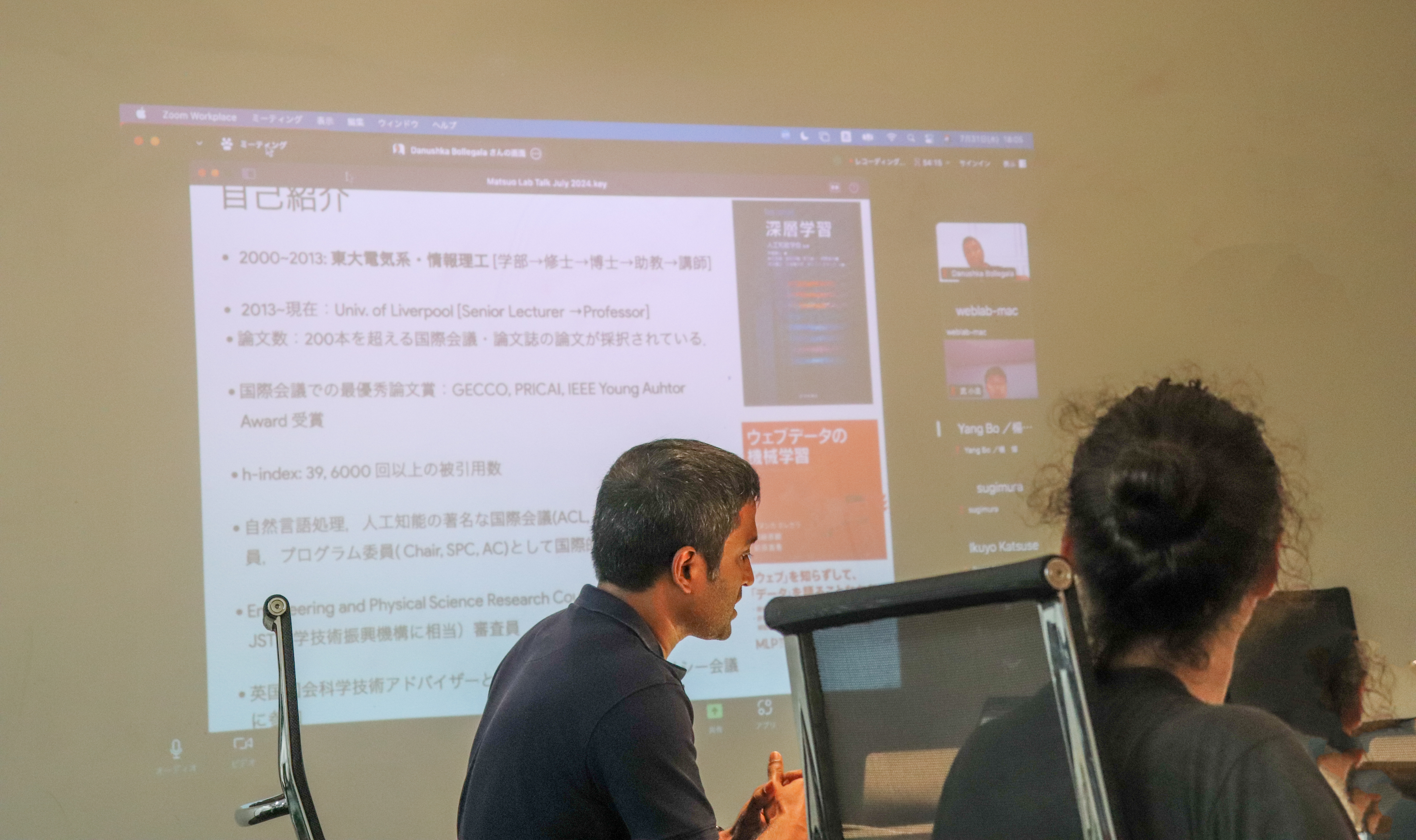
Speaker biography:.
Danushka Bollegala is a Professor in Natural Language Processing (NLP) at the Department of Computer Science, The University of Liverpool, United Kingdom. He leads the Machine Learning (ML) and NLP Research Groups. He is also an Amazon Scholar working at the cutting-edge information retrieval problems at Amazon Search. He obtains his PhD in 2019 from the University of Tokyo and worked as a lecturer in the Graduate School of Information Science and He has published over 200 peer-reviewed articles including top venues in NLP/AI such as ACL, EMNLP, IJCAI and AAAI. is the author of two popular Japanese text books on Deep Learning and its application to text mining.
Title: Towards the LLM that reads the air
Abstract: The following is a list of the most common types of
Large Language Models (LLMs) have revolutionised the way we access information. However, the responses returned by the LLMs are agnostic to the human users’ background and thus remain less diverse. In this talk, I will present our latest research work on diversifying natural As a concrete example, I will focus on Generative Commonsense Reasoning (GCR), where given a set of input concepts (e.g. dog, catch, frisbee, throw), the response returned by the LLMs are agnostic to the human users’ background and thus remain less diverse. catch, frisbee, throw), a generative model is required to generate a commonsense bearing sentence that covers all of the input concepts (e.g. The dog caught the frisbee thrown at it). I will show that simple heuristics such as increasing the sampling temperature leads to poor generations that artificially inflate diversity metrics. As a solution, I will present an incontext learning-based approach to improve diversity in GCR, while maintaining the generation quality.

Several students in the Matsuo Lab are involved in joint research with overseas researchers, and there are opportunities to actively exchange opinions with people outside the laboratory. Several students in the Matsuo Lab are involved in joint research with overseas researchers, and there are opportunities to actively exchange opinions with people outside the laboratory.
We hope to continue this kind of active exchange across laboratory boundaries in the future.
Prof. Danushka, thank you very much for visiting the Matsuo Lab.